Empowering AI in Manufacturing: Augmenting Human Expertise
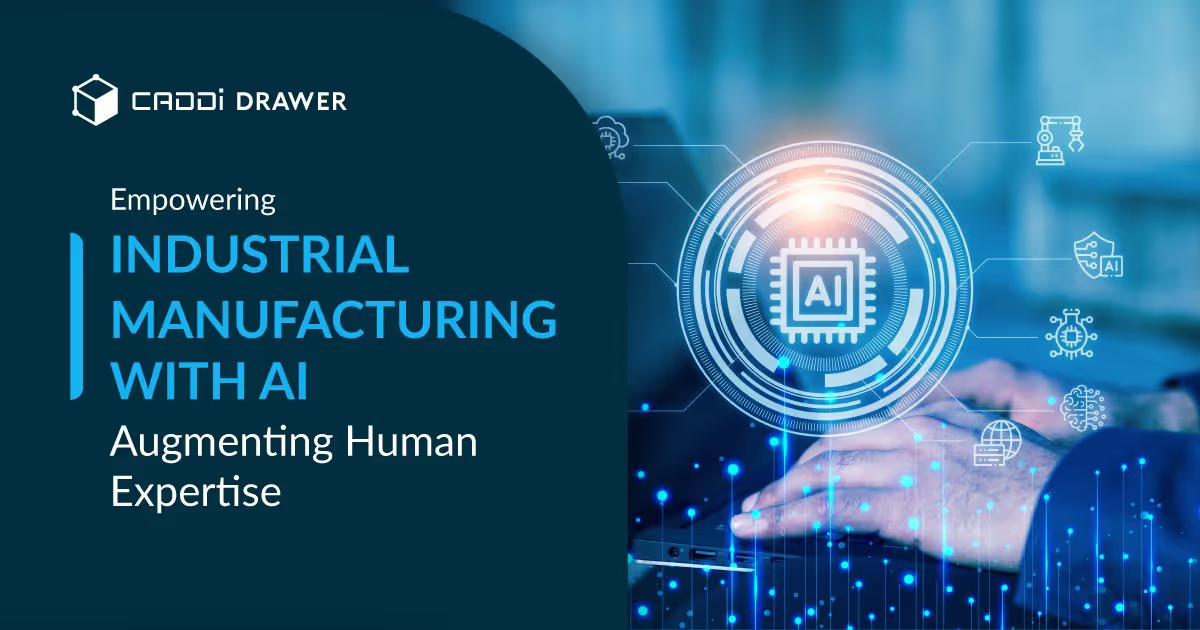
Table of Contents
In the rapidly evolving landscape of the manufacturing industry, the integration of Artificial Intelligence (AI) stands as a beacon of potential transformation. Yet, despite its promise, there remains a significant gap in the application of AI in manufacturing compared to other sectors. This disparity not only highlights a missed opportunity for efficiency and innovation but also underscores the need for a strategic reevaluation of how AI can serve the industry.
The Landscape of AI in Manufacturing: Identifying Gaps
The application of AI and digital technologies in manufacturing still lags behind other industries, creating a noticeable gap. According to a Deloitte survey on AI in manufacturing, while 93% of companies believe AI will be crucial for growth and innovation in the sector, only a limited number have deployed AI in products and services so far. The McKinsey report also highlights that AI adoption remains low in the industrial sector, despite the potential for significant economic value. In response to the shortage of skilled workers, many manufacturers are turning to AI to improve productivity and efficiency. 85% of US manufacturers planning to adopt or have already adopted AI according to Fictiv’s report on state of manufacturing 2023.
Key challenges across the manufacturing value chain
The challenges in the supply chain faced by the manufacturing industry primarily include quality improvement, cost reduction, expansion of manufacturing capacity, and securing a stable supply system from suppliers. By breaking down the value chain into steps such as design, cost planning, procurement, and production, we can identify specific challenges at each stage. Here are some major challenges especially in the machinery manufacturing space.
Design and Engineering
Searchability of relevant data: The visibility and searchability of past data, especially including drawings, price information, and quality information, are not always high. This leads to the creation of many new drawings and an increase in design workload and chances of “reinventing wheels”.
Coordination with Manufacturing: There’s a lack of necessary information in design drawings and related documents for manufacturing processes. As a result, drawings that do not sufficiently consider manufacturability are created, leading to cases of quality defects.
High Volume of Inquiries: There are many inquiries related to design and engineering, from suppliers, production and some other departments most of which stem from deficiencies and inconsistencies in the drawings.
Cost Planning
Accuracy of Theoretical Cost Calculation: Although theoretical cost calculation software exists, it’s difficult to fully reflect numerous fluctuating factors such as material prices, variance in supplier estimates, and macroeconomic factors, leading to significant discrepancies between the theoretical and actual prices. There is uncertainty in calculating design unit prices based on the actual prices of similar drawings.
Difficulty in Cost Reduction Measures: Efforts are made to consider fundamental approaches to cost reduction such as VAVE, and supplier consolidation. Despite that, it's challenging to complete the analysis to action due to the required workload and level of expertise.
Procurement
Supplier Management: Managing a large number of suppliers, despite being small-scale, is challenging, especially for manufacturers in High-mix Low volume space.
Trend of Rising Costs: There is a continuous trend of significant price increases in material and transportation costs.
New Supplier Discovery: The cost of searching for new suppliers to fill capacity shortages is high.
Production
Manufacturing Control: Responding to process changes is difficult, and the criteria for deciding between in-house and outsourced production are unclear.
Inventory Management: Inventory tracking and visualization are challenging, leading to problems with shortages or excess inventory.
Practical Applications of AI in Machinery Manufacturing: A Balanced Approach
The application of AI in manufacturing is vast and varied, encompassing predictive maintenance, quality control, supply chain optimization, and beyond. However, it’s crucial to approach these applications with a balanced view, recognizing the areas where AI in manufacturing can improve efficiency and product quality, while also acknowledging the irreplaceable value of human expertise. For instance, while AI can predict machine failure and schedule maintenance, the nuanced judgment of an experienced technician in interpreting these predictions remains paramount.
Design and Engineering
In machine manufacturing, AI optimizes product design, increases innovation, and improves efficiency in design and engineering. For example, AI is being used for generative design, simulation, and virtual testing to create more efficient and cost-effective machinery. Engineering software such as Autodesk and Solidworks have implemented AI-powered features to reduce the time spent on non-value-added tasks and enable engineers and designers to focus on core engineering tasks, including new product design and product development.
Cost Planning
AI can be helpful for cost planning and the work of cost modeling. As cost modeling involves numerous variables and data points to calculate the theoretical cost for machine products, AI’s capability to gather, analyze, and process data through machine learning technologies assists engineering and procurement teams in
Procurement
In machinery manufacturing, lots of AI tools swiftly find and engage with alternative suppliers during unexpected disruptions. These tools help streamline the procurement process by pre-qualifying suppliers and providing valuable information for securing alternate sources of supplies for machinery production.
Manufacturing
AI plays a significant role in machinery manufacturing by enhancing efficiency, boosting quality, and revolutionizing processes. Some applications include predictive maintenance to reduce downtime, product quality inspection using AI-powered vision systems, and supply chain optimization for improved efficiency and cost-effectiveness specifically in the context of machinery production.
Need for cross-sectional collaboration and democratization of tribal knowledge for AI in manufacturing
Many of these challenges cannot be solved by a single organization or approach alone; neither digital nor traditional solutions can address them independently. Building a data foundation and facilitating communication through the collaboration of multiple organizations is essential.
Generally, these challenges are treated as design issues. CAD vendors provide systems or departments implement improvements. However, they primarily arise between the simulation in “design” and the reality of “parts and finished products”.
Understanding the issues of design quality and the need for VAVE (Value Analysis/Value Engineering) for cost reduction is possible only with knowledge of real manufacturing and design information. From this perspective, procurement, positioned between “design information” and “actual manufacturing” and connecting them while holding critical Quality, Cost, and Delivery (QCD) information, is clearly the suitable hub and starting point for addressing this problem.
In addition, many overlook that a lot of critical data to improve manufacturing is stored as unstructured data, such as legacy drawings on paper or scanned PDFs. Additionally, handwritten notes and the tribal knowledge stored in the heads of experienced engineers and procurement leaders who have been with the company for 30 years are invaluable. AI cannot generate or replace this knowledge. Manufacturers require the ability to transform this scattered knowledge and data into assets in a form that can be accessed, analyzed, and utilized by anyone who needs that information.
How CADDi Enhances Human Expertise leveraging AI
In line with our commitment to enhancing human expertise with AI, CADDi offers a suite of solutions designed to empower manufacturing employees. We develop our tools with the dual goals of optimizing manufacturing processes and augmenting the capabilities of the workforce. Check out the capabilities of CADDi Drawer to facilitate cross-sectional collaboration and democratize tribal knowledge.
Conclusion: The Future of Manufacturing with AI
As we look towards the future, it’s clear that the integration of AI in manufacturing presents a pivotal opportunity for the industry. However, the success of this integration hinges on a collective commitment to viewing AI as a tool to empower and enhance human capabilities, not as a replacement. By embracing AI in this spirit, manufacturers can not only bridge the current gap in technology adoption but also pave the way for a new era of innovation and growth.
For C-suite executives in the manufacturing sector, the message is clear: the strategic implementation of AI solutions offers a path to not only achieving operational excellence but also to fostering a more skilled, satisfied, and innovative workforce. As we continue to navigate the complexities of the manufacturing landscape, let us do so with the conviction that AI and human expertise together hold the key to unlocking the full potential of the industry.
In the rapidly evolving landscape of the manufacturing industry, the integration of Artificial Intelligence (AI) stands as a beacon of potential transformation. Yet, despite its promise, there remains a significant gap in the application of AI in manufacturing compared to other sectors. This disparity not only highlights a missed opportunity for efficiency and innovation but also underscores the need for a strategic reevaluation of how AI can serve the industry.
The Landscape of AI in Manufacturing: Identifying Gaps
The application of AI and digital technologies in manufacturing still lags behind other industries, creating a noticeable gap. According to a Deloitte survey on AI in manufacturing, while 93% of companies believe AI will be crucial for growth and innovation in the sector, only a limited number have deployed AI in products and services so far. The McKinsey report also highlights that AI adoption remains low in the industrial sector, despite the potential for significant economic value. In response to the shortage of skilled workers, many manufacturers are turning to AI to improve productivity and efficiency. 85% of US manufacturers planning to adopt or have already adopted AI according to Fictiv’s report on state of manufacturing 2023.
Key challenges across the manufacturing value chain
The challenges in the supply chain faced by the manufacturing industry primarily include quality improvement, cost reduction, expansion of manufacturing capacity, and securing a stable supply system from suppliers. By breaking down the value chain into steps such as design, cost planning, procurement, and production, we can identify specific challenges at each stage. Here are some major challenges especially in the machinery manufacturing space.
Design and Engineering
Searchability of relevant data: The visibility and searchability of past data, especially including drawings, price information, and quality information, are not always high. This leads to the creation of many new drawings and an increase in design workload and chances of “reinventing wheels”.
Coordination with Manufacturing: There’s a lack of necessary information in design drawings and related documents for manufacturing processes. As a result, drawings that do not sufficiently consider manufacturability are created, leading to cases of quality defects.
High Volume of Inquiries: There are many inquiries related to design and engineering, from suppliers, production and some other departments most of which stem from deficiencies and inconsistencies in the drawings.
Cost Planning
Accuracy of Theoretical Cost Calculation: Although theoretical cost calculation software exists, it’s difficult to fully reflect numerous fluctuating factors such as material prices, variance in supplier estimates, and macroeconomic factors, leading to significant discrepancies between the theoretical and actual prices. There is uncertainty in calculating design unit prices based on the actual prices of similar drawings.
Difficulty in Cost Reduction Measures: Efforts are made to consider fundamental approaches to cost reduction such as VAVE, and supplier consolidation. Despite that, it's challenging to complete the analysis to action due to the required workload and level of expertise.
Procurement
Supplier Management: Managing a large number of suppliers, despite being small-scale, is challenging, especially for manufacturers in High-mix Low volume space.
Trend of Rising Costs: There is a continuous trend of significant price increases in material and transportation costs.
New Supplier Discovery: The cost of searching for new suppliers to fill capacity shortages is high.
Production
Manufacturing Control: Responding to process changes is difficult, and the criteria for deciding between in-house and outsourced production are unclear.
Inventory Management: Inventory tracking and visualization are challenging, leading to problems with shortages or excess inventory.
Practical Applications of AI in Machinery Manufacturing: A Balanced Approach
The application of AI in manufacturing is vast and varied, encompassing predictive maintenance, quality control, supply chain optimization, and beyond. However, it’s crucial to approach these applications with a balanced view, recognizing the areas where AI in manufacturing can improve efficiency and product quality, while also acknowledging the irreplaceable value of human expertise. For instance, while AI can predict machine failure and schedule maintenance, the nuanced judgment of an experienced technician in interpreting these predictions remains paramount.
Design and Engineering
In machine manufacturing, AI optimizes product design, increases innovation, and improves efficiency in design and engineering. For example, AI is being used for generative design, simulation, and virtual testing to create more efficient and cost-effective machinery. Engineering software such as Autodesk and Solidworks have implemented AI-powered features to reduce the time spent on non-value-added tasks and enable engineers and designers to focus on core engineering tasks, including new product design and product development.
Cost Planning
AI can be helpful for cost planning and the work of cost modeling. As cost modeling involves numerous variables and data points to calculate the theoretical cost for machine products, AI’s capability to gather, analyze, and process data through machine learning technologies assists engineering and procurement teams in
Procurement
In machinery manufacturing, lots of AI tools swiftly find and engage with alternative suppliers during unexpected disruptions. These tools help streamline the procurement process by pre-qualifying suppliers and providing valuable information for securing alternate sources of supplies for machinery production.
Manufacturing
AI plays a significant role in machinery manufacturing by enhancing efficiency, boosting quality, and revolutionizing processes. Some applications include predictive maintenance to reduce downtime, product quality inspection using AI-powered vision systems, and supply chain optimization for improved efficiency and cost-effectiveness specifically in the context of machinery production.
Need for cross-sectional collaboration and democratization of tribal knowledge for AI in manufacturing
Many of these challenges cannot be solved by a single organization or approach alone; neither digital nor traditional solutions can address them independently. Building a data foundation and facilitating communication through the collaboration of multiple organizations is essential.
Generally, these challenges are treated as design issues. CAD vendors provide systems or departments implement improvements. However, they primarily arise between the simulation in “design” and the reality of “parts and finished products”.
Understanding the issues of design quality and the need for VAVE (Value Analysis/Value Engineering) for cost reduction is possible only with knowledge of real manufacturing and design information. From this perspective, procurement, positioned between “design information” and “actual manufacturing” and connecting them while holding critical Quality, Cost, and Delivery (QCD) information, is clearly the suitable hub and starting point for addressing this problem.
In addition, many overlook that a lot of critical data to improve manufacturing is stored as unstructured data, such as legacy drawings on paper or scanned PDFs. Additionally, handwritten notes and the tribal knowledge stored in the heads of experienced engineers and procurement leaders who have been with the company for 30 years are invaluable. AI cannot generate or replace this knowledge. Manufacturers require the ability to transform this scattered knowledge and data into assets in a form that can be accessed, analyzed, and utilized by anyone who needs that information.
How CADDi Enhances Human Expertise leveraging AI
In line with our commitment to enhancing human expertise with AI, CADDi offers a suite of solutions designed to empower manufacturing employees. We develop our tools with the dual goals of optimizing manufacturing processes and augmenting the capabilities of the workforce. Check out the capabilities of CADDi Drawer to facilitate cross-sectional collaboration and democratize tribal knowledge.
Conclusion: The Future of Manufacturing with AI
As we look towards the future, it’s clear that the integration of AI in manufacturing presents a pivotal opportunity for the industry. However, the success of this integration hinges on a collective commitment to viewing AI as a tool to empower and enhance human capabilities, not as a replacement. By embracing AI in this spirit, manufacturers can not only bridge the current gap in technology adoption but also pave the way for a new era of innovation and growth.
For C-suite executives in the manufacturing sector, the message is clear: the strategic implementation of AI solutions offers a path to not only achieving operational excellence but also to fostering a more skilled, satisfied, and innovative workforce. As we continue to navigate the complexities of the manufacturing landscape, let us do so with the conviction that AI and human expertise together hold the key to unlocking the full potential of the industry.
Ready to see CADDi Drawer in action? Get a personalized demo.
Related Resources


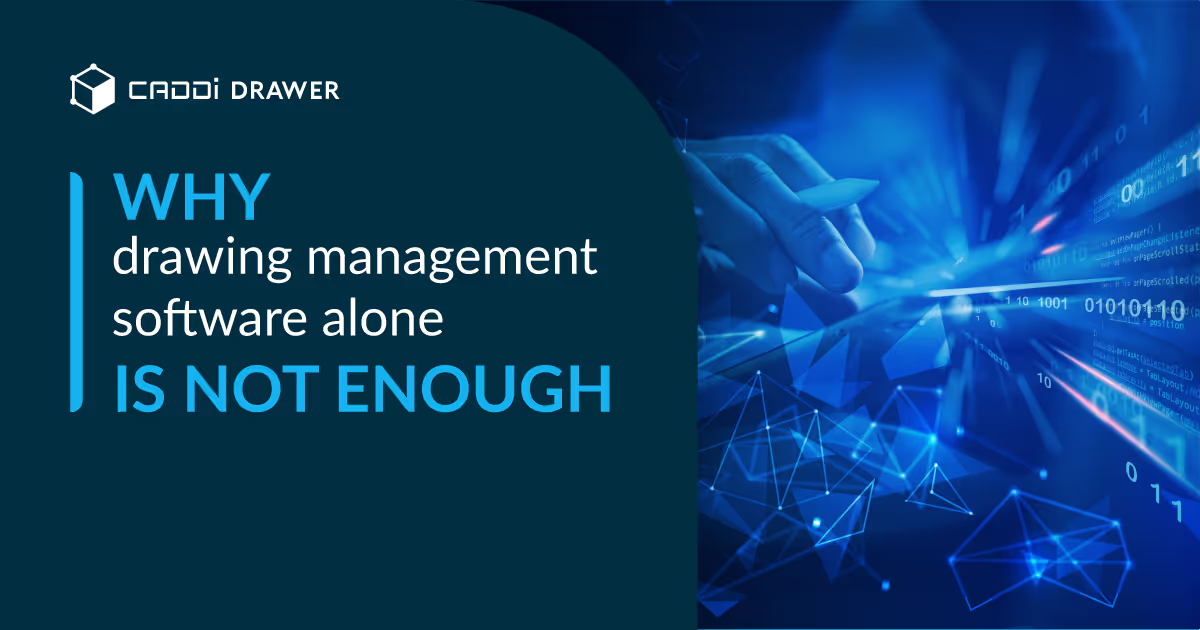

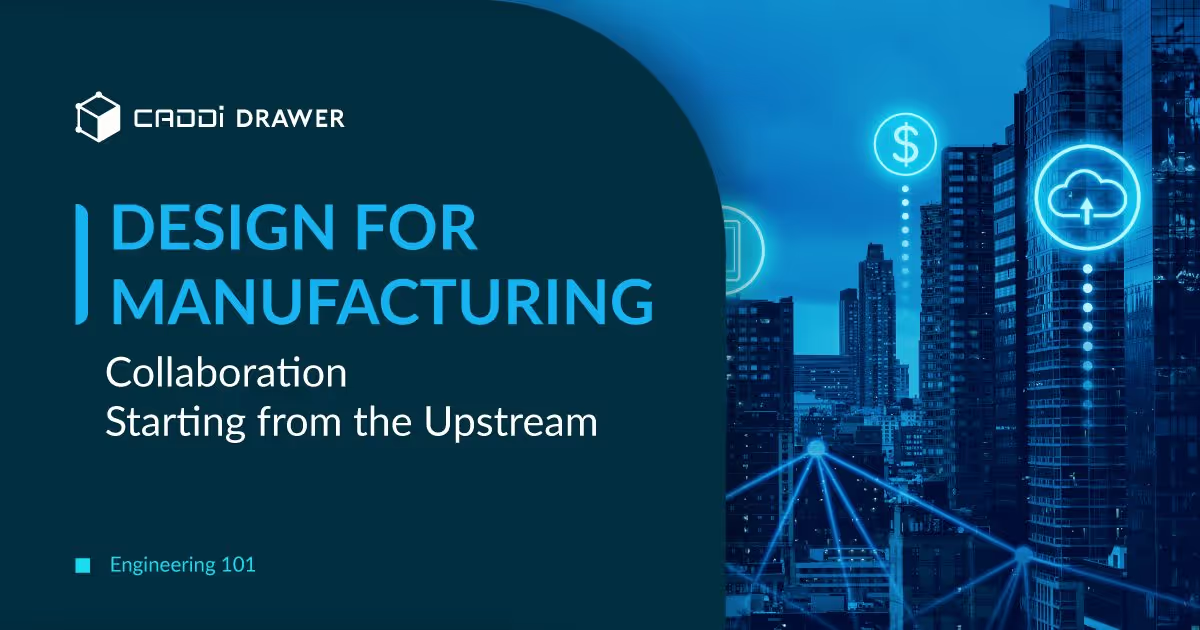