Quality Management System evolving through advanced data management
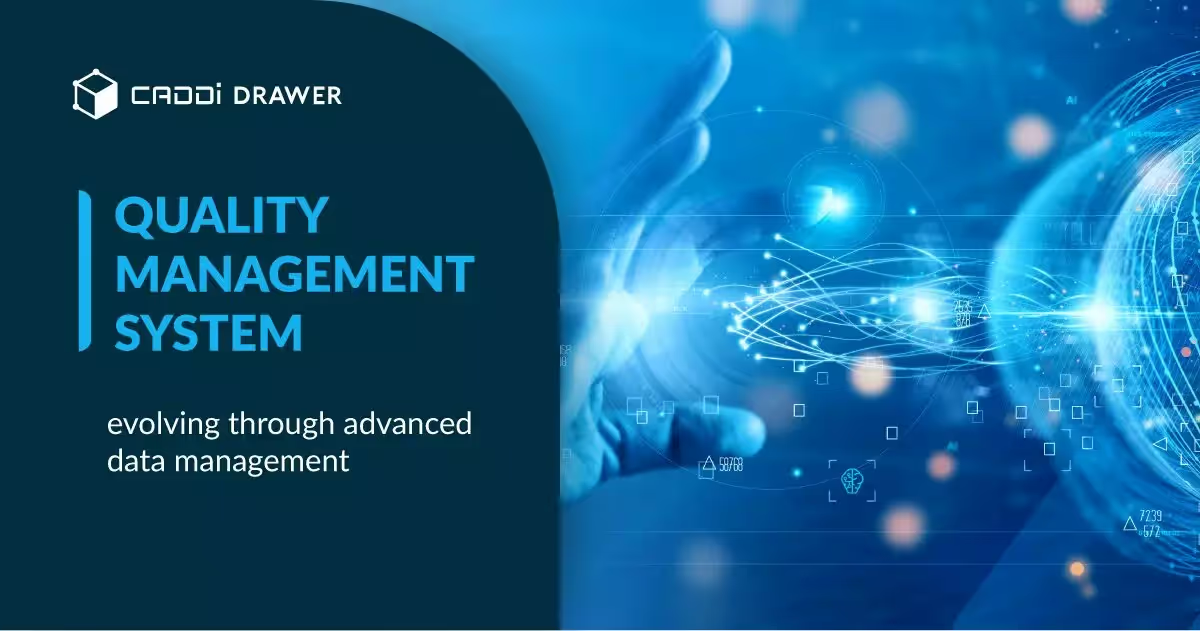
Table of Contents
Gone are the days of inefficient processes, inconsistent quality, and frustrated customers. Today, forward-thinking manufacturers are harnessing the might of data to revolutionize the way they operate, and the results are nothing short of extraordinary. From reducing defects and waste to enhancing efficiency and customer loyalty, the benefits of a data-driven Quality Management System (QMS) are clear. But how exactly are these systems evolving, and what does the future hold for quality management in the digital age?
The Foundation of Quality Management Systems
A QMS is a formalized system that documents processes, procedures, and responsibilities for achieving quality policies and objectives. It is a comprehensive approach to ensuring that an organization consistently meets and exceeds customer requirements and regulatory standards.
Key components of a QMS include:
- Quality Policy and Objectives: A clear statement of the organization’s commitment to quality and the specific objectives that will be pursued to achieve it.
- Quality Manual: A document that describes the QMS and how it applies to the organization’s activities.
- Organizational Structure and Responsibilities: A clear definition of roles, responsibilities, and authorities for managing, performing, and verifying work affecting quality.
- Data Management: Procedures for identifying, collecting, indexing, accessing, filing, storing, maintaining, and disposing of quality records.
- Processes: A set of interrelated or interacting activities that transform inputs into outputs. Processes should be documented, controlled, and continuously improved.
- Customer Focus: A strong emphasis on understanding and meeting customer requirements and expectations.
- Resource Management: Procedures for identifying and providing the resources needed to implement and maintain the QMS.
- Product Realization: Procedures for planning and developing the processes needed for product realization, including design and development, purchasing, production and service provision, and control of monitoring and measuring devices.
- Measurement, Analysis, and Improvement: Procedures for monitoring, measuring, analyzing, and improving the QMS and the products and services it produces.
- Continuous Improvement: A commitment to continuously improving the effectiveness of the QMS through the use of quality objectives, audit results, data analysis, corrective and preventive actions, and management review.
These components work together to ensure that an organization has a systematic approach to quality management, leading to consistent and reliable products and services, satisfied customers, and a culture of continuous improvement.
However, according to a study by ASQ, the cost of poor quality can range from 15% to 40% of an organization’s revenue. This includes expenses related to defects, rework, warranty claims, and lost customers. By addressing the challenges of traditional QMS, businesses can significantly reduce these costs and improve their bottom line.
Documents and Data in Quality Management Systems
Running a QMS relies heavily on various documents and data to ensure effective operation and continuous improvement.
Some of the key documents and data needed in a QMS include:
- Quality Policy and Objectives: Written statements that define the organization’s commitment to quality and specific goals to be achieved.
- Quality Manual: A document that describes the overall QMS, including its scope, processes, and procedures.
- Standard Operating Procedures (SOPs): Detailed instructions for carrying out specific processes or tasks.
- Work Instructions: Step-by-step guides for performing specific operations or using equipment.
- Quality Reports and Templates: Standardized documents used to record data, such as inspection reports, audit checklists, and corrective action requests.
- Product Specifications: Detailed descriptions of the characteristics, features, and requirements of a product or service.
- Training Records: Documentation of employee training, including dates, topics covered, and certifications earned.
- Audit Reports: Results of internal and external audits, including findings, observations, and recommendations for improvement.
- Customer Feedback: Data collected from customers, such as satisfaction surveys, complaint records, and warranty claims.
- Performance Metrics: Measurable data that track the effectiveness and efficiency of processes, such as cycle time, defect rates, and on-time delivery.
Data management is crucial in a QMS for several reasons:
- Decision Making: Accurate and timely data allows organizations to make informed decisions about quality improvement initiatives, resource allocation, and strategic planning.
- Traceability: Effective data management ensures that products and processes can be traced throughout the supply chain, enabling faster problem resolution and recall management.
- Compliance: Many industries have specific regulatory requirements for data management, such as data retention periods and access controls.
- Continuous Improvement: By analyzing trends and patterns in quality data, organizations can identify opportunities for improvement and measure the effectiveness of quality initiatives.
Challenges in Data Management:
Despite its importance, data management in QMS processes can present several challenges:
Data Silos: Quality data may be spread across various systems and departments, making it difficult to access and analyze in a holistic manner.
Data Quality: Ensuring the accuracy, completeness, and consistency of quality data can be challenging, particularly when manual data entry is involved. Relying on manual data entry and analysis can lead to mistakes, delays, and inconsistencies.
Data Integration: Integrating quality data from multiple sources, such as ERP systems, manufacturing execution systems, and customer relationship management systems, can be complex and time-consuming.
Data Volume: As organizations collect more quality data from various sources, managing and analyzing large volumes of data can become increasingly difficult.
Limited Visibility and Insight: Without real-time data and advanced analytics, organizations may struggle to identify trends, patterns, and opportunities for improvement.
To address these challenges, organizations are turning to advanced data management technologies, such as cloud-based platforms, data lakes, and artificial intelligence tools. By leveraging these technologies, organizations can break down data silos, improve data quality, automate data integration, and gain real-time insights into quality performance. However, successful data management also requires a strong commitment to data governance, including clear policies, procedures, and responsibilities for managing quality data throughout its lifecycle.
The Data Revolution in Quality Management
The rise of advanced data management technologies is transforming the way organizations approach quality management. By leveraging the power of Big Data, the Industrial IoT, and AI, manufacturers can gain unprecedented visibility into their operations and make data-driven decisions that drive continuous improvement.
Real-Time Monitoring and Analysis
One of the key benefits of advanced data management in QMS is the ability to monitor and analyze quality data in real-time. By using sensors, IoT devices, and cloud-based platforms, manufacturers can track every aspect of their production processes, from raw material intake to final product delivery. This allows them to quickly identify and address quality issues, reduce downtime, and optimize performance.
Predictive Quality Management
Another exciting development in data-driven QMS is the use of predictive analytics and machine learning algorithms. By analyzing vast amounts of historical data, these intelligent systems can identify patterns and anomalies that may indicate potential quality issues. This allows manufacturers to take proactive measures to prevent defects and failures before they occur.
Beyond Technology: The Human Factor in Quality Management
While advanced data management and cutting-edge technologies are essential components of a modern QMS, they are not the only factors that contribute to success. To truly achieve excellence in quality management, organizations must also focus on the human aspects of their operations.
Reviewing and Standardizing Business Processes
One of the key steps in improving quality management is to carefully review and standardize business processes. This involves mapping out the current state of operations, identifying inefficiencies and bottlenecks, and developing standardized procedures that ensure consistency and reliability.
By creating a clear and well-documented set of processes, organizations can reduce variability, minimize errors, and improve overall quality. This also makes it easier to train new employees, as they have a clear roadmap to follow and understand their roles and responsibilities.
Changing Organizational Culture and Evaluation Systems
Another critical aspect of effective quality management is fostering a culture of quality throughout the organization. This means moving beyond simply meeting minimum standards and instead striving for continuous improvement and excellence.
To achieve this, organizations must align their evaluation systems and incentives with their quality goals. This may involve:
- Setting clear quality metrics and KPIs
- Tying performance evaluations and bonuses to quality outcomes
- Recognizing and rewarding employees who demonstrate a commitment to quality
- Encouraging open communication and collaboration across departments
By creating a culture where quality is everyone’s responsibility and is consistently prioritized, organizations can drive meaningful and lasting change.
Investing in Training and Employee Development
Finally, investing in training and employee development is crucial for maintaining a high level of quality. As technologies and best practices evolve, it’s essential that employees have the skills and knowledge they need to adapt and excel.
This may involve:
- Providing regular training and workshops on quality management techniques
- Encouraging employees to pursue certifications and continuing education opportunities
- Fostering a culture of learning and knowledge sharing
- Empowering employees to take ownership of quality in their work
By investing in their people and providing them with the tools and resources they need to succeed, organizations can build a strong foundation for quality management excellence.
Case Study: IBM’s Cognitive QMS
IBM’s Cognitive QMS, powered by Watson AI, is a prime example of how advanced data management can revolutionize quality management. This innovative system integrates with existing QMS processes and provides intelligent insights to help manufacturers optimize quality, efficiency, and compliance.
Key Features and Benefits
Cognitive Process Optimization: Watson AI analyzes vast amounts of structured and unstructured data to identify patterns, anomalies, and opportunities for improvement.
Predictive Quality Insights: By leveraging machine learning algorithms, the system can predict potential quality issues and provide recommendations for preventive action.
Compliance and Risk Management: IBM’s Cognitive QMS helps manufacturers ensure compliance with industry standards and regulations, while also identifying and mitigating potential risks.
Real-World Results
In a case study published by IBM, a leading electronics manufacturer implemented Cognitive QMS and achieved remarkable results:
- 25% reduction in defects
- 15% improvement in overall equipment effectiveness
- 30% reduction in quality management costs
Overcoming Obstacles and Achieving Success
Implementing a data-driven Quality Management System is not without its challenges. Some common obstacles organizations may face include:
- Resistance to change: Employees may be hesitant to adopt new technologies or processes, particularly if they are comfortable with the status quo.
- Integration issues: Integrating new systems with existing infrastructure can be complex and time-consuming.
- Data quality concerns: Ensuring the accuracy, completeness, and reliability of data is essential for effective decision-making.
To overcome these obstacles and achieve success, organizations must:
- Start small and scale gradually: Begin with pilot projects or targeted implementations, and gradually expand as success is demonstrated and lessons are learned.
- Provide adequate training and support: Ensure that employees have the skills and resources they need to effectively use new technologies and processes.
- Continuously monitor and optimize: Regularly assess the performance of the QMS, and make adjustments as needed to ensure ongoing effectiveness and efficiency.
How CADDi Drawer Can Help in the QMS
The future of QMS is bright, and the potential for transformation is limitless. By leveraging the power of advanced data management, fostering a culture of continuous improvement, and investing in the development of their people, manufacturers can achieve new levels of quality, efficiency, and customer satisfaction.
CADDi Drawer is one of the solutions that support such data management. By using this solution, you can:
- Centralized management of documents and data: It breaks down siloed data structures and allows you to link supplier data, purchasing data, quality data, and various document groups related to QMS by using drawings, which are a shared medium throughout the manufacturing value chain, as a key.
- Make information in documents and drawings searchable: With its unique OCR algorithm, it can read and keyword-search text within documents such as SOPs, Work Instructions, Quality reports, and even footnotes in drawings.

These features significantly reduce the time spent searching for past data, allowing you to focus more on essential tasks. It also prevents overlooking necessary information or past lessons and missing out on collaboration. By doing so, it ensures the reliable execution of continuous improvement.
As you embark on your own journey to revolutionize your QMS, remember that success is not just about adopting new technologies or processes. It’s about fundamentally changing the way you think about quality and embracing a culture of excellence.
So, ask yourself:
- What steps can you take today to harness the power of data and drive meaningful change in your organization?
- How can you empower your employees to take ownership of quality and continuously improve?
- What investments can you make in training and development to build a strong foundation for success?
The answers to these questions will guide you on your path to a more intelligent, adaptive, and effective Quality Management System. The future is here, and the time to act is now.
Gone are the days of inefficient processes, inconsistent quality, and frustrated customers. Today, forward-thinking manufacturers are harnessing the might of data to revolutionize the way they operate, and the results are nothing short of extraordinary. From reducing defects and waste to enhancing efficiency and customer loyalty, the benefits of a data-driven Quality Management System (QMS) are clear. But how exactly are these systems evolving, and what does the future hold for quality management in the digital age?
The Foundation of Quality Management Systems
A QMS is a formalized system that documents processes, procedures, and responsibilities for achieving quality policies and objectives. It is a comprehensive approach to ensuring that an organization consistently meets and exceeds customer requirements and regulatory standards.
Key components of a QMS include:
- Quality Policy and Objectives: A clear statement of the organization’s commitment to quality and the specific objectives that will be pursued to achieve it.
- Quality Manual: A document that describes the QMS and how it applies to the organization’s activities.
- Organizational Structure and Responsibilities: A clear definition of roles, responsibilities, and authorities for managing, performing, and verifying work affecting quality.
- Data Management: Procedures for identifying, collecting, indexing, accessing, filing, storing, maintaining, and disposing of quality records.
- Processes: A set of interrelated or interacting activities that transform inputs into outputs. Processes should be documented, controlled, and continuously improved.
- Customer Focus: A strong emphasis on understanding and meeting customer requirements and expectations.
- Resource Management: Procedures for identifying and providing the resources needed to implement and maintain the QMS.
- Product Realization: Procedures for planning and developing the processes needed for product realization, including design and development, purchasing, production and service provision, and control of monitoring and measuring devices.
- Measurement, Analysis, and Improvement: Procedures for monitoring, measuring, analyzing, and improving the QMS and the products and services it produces.
- Continuous Improvement: A commitment to continuously improving the effectiveness of the QMS through the use of quality objectives, audit results, data analysis, corrective and preventive actions, and management review.
These components work together to ensure that an organization has a systematic approach to quality management, leading to consistent and reliable products and services, satisfied customers, and a culture of continuous improvement.
However, according to a study by ASQ, the cost of poor quality can range from 15% to 40% of an organization’s revenue. This includes expenses related to defects, rework, warranty claims, and lost customers. By addressing the challenges of traditional QMS, businesses can significantly reduce these costs and improve their bottom line.
Documents and Data in Quality Management Systems
Running a QMS relies heavily on various documents and data to ensure effective operation and continuous improvement.
Some of the key documents and data needed in a QMS include:
- Quality Policy and Objectives: Written statements that define the organization’s commitment to quality and specific goals to be achieved.
- Quality Manual: A document that describes the overall QMS, including its scope, processes, and procedures.
- Standard Operating Procedures (SOPs): Detailed instructions for carrying out specific processes or tasks.
- Work Instructions: Step-by-step guides for performing specific operations or using equipment.
- Quality Reports and Templates: Standardized documents used to record data, such as inspection reports, audit checklists, and corrective action requests.
- Product Specifications: Detailed descriptions of the characteristics, features, and requirements of a product or service.
- Training Records: Documentation of employee training, including dates, topics covered, and certifications earned.
- Audit Reports: Results of internal and external audits, including findings, observations, and recommendations for improvement.
- Customer Feedback: Data collected from customers, such as satisfaction surveys, complaint records, and warranty claims.
- Performance Metrics: Measurable data that track the effectiveness and efficiency of processes, such as cycle time, defect rates, and on-time delivery.
Data management is crucial in a QMS for several reasons:
- Decision Making: Accurate and timely data allows organizations to make informed decisions about quality improvement initiatives, resource allocation, and strategic planning.
- Traceability: Effective data management ensures that products and processes can be traced throughout the supply chain, enabling faster problem resolution and recall management.
- Compliance: Many industries have specific regulatory requirements for data management, such as data retention periods and access controls.
- Continuous Improvement: By analyzing trends and patterns in quality data, organizations can identify opportunities for improvement and measure the effectiveness of quality initiatives.
Challenges in Data Management:
Despite its importance, data management in QMS processes can present several challenges:
Data Silos: Quality data may be spread across various systems and departments, making it difficult to access and analyze in a holistic manner.
Data Quality: Ensuring the accuracy, completeness, and consistency of quality data can be challenging, particularly when manual data entry is involved. Relying on manual data entry and analysis can lead to mistakes, delays, and inconsistencies.
Data Integration: Integrating quality data from multiple sources, such as ERP systems, manufacturing execution systems, and customer relationship management systems, can be complex and time-consuming.
Data Volume: As organizations collect more quality data from various sources, managing and analyzing large volumes of data can become increasingly difficult.
Limited Visibility and Insight: Without real-time data and advanced analytics, organizations may struggle to identify trends, patterns, and opportunities for improvement.
To address these challenges, organizations are turning to advanced data management technologies, such as cloud-based platforms, data lakes, and artificial intelligence tools. By leveraging these technologies, organizations can break down data silos, improve data quality, automate data integration, and gain real-time insights into quality performance. However, successful data management also requires a strong commitment to data governance, including clear policies, procedures, and responsibilities for managing quality data throughout its lifecycle.
The Data Revolution in Quality Management
The rise of advanced data management technologies is transforming the way organizations approach quality management. By leveraging the power of Big Data, the Industrial IoT, and AI, manufacturers can gain unprecedented visibility into their operations and make data-driven decisions that drive continuous improvement.
Real-Time Monitoring and Analysis
One of the key benefits of advanced data management in QMS is the ability to monitor and analyze quality data in real-time. By using sensors, IoT devices, and cloud-based platforms, manufacturers can track every aspect of their production processes, from raw material intake to final product delivery. This allows them to quickly identify and address quality issues, reduce downtime, and optimize performance.
Predictive Quality Management
Another exciting development in data-driven QMS is the use of predictive analytics and machine learning algorithms. By analyzing vast amounts of historical data, these intelligent systems can identify patterns and anomalies that may indicate potential quality issues. This allows manufacturers to take proactive measures to prevent defects and failures before they occur.
Beyond Technology: The Human Factor in Quality Management
While advanced data management and cutting-edge technologies are essential components of a modern QMS, they are not the only factors that contribute to success. To truly achieve excellence in quality management, organizations must also focus on the human aspects of their operations.
Reviewing and Standardizing Business Processes
One of the key steps in improving quality management is to carefully review and standardize business processes. This involves mapping out the current state of operations, identifying inefficiencies and bottlenecks, and developing standardized procedures that ensure consistency and reliability.
By creating a clear and well-documented set of processes, organizations can reduce variability, minimize errors, and improve overall quality. This also makes it easier to train new employees, as they have a clear roadmap to follow and understand their roles and responsibilities.
Changing Organizational Culture and Evaluation Systems
Another critical aspect of effective quality management is fostering a culture of quality throughout the organization. This means moving beyond simply meeting minimum standards and instead striving for continuous improvement and excellence.
To achieve this, organizations must align their evaluation systems and incentives with their quality goals. This may involve:
- Setting clear quality metrics and KPIs
- Tying performance evaluations and bonuses to quality outcomes
- Recognizing and rewarding employees who demonstrate a commitment to quality
- Encouraging open communication and collaboration across departments
By creating a culture where quality is everyone’s responsibility and is consistently prioritized, organizations can drive meaningful and lasting change.
Investing in Training and Employee Development
Finally, investing in training and employee development is crucial for maintaining a high level of quality. As technologies and best practices evolve, it’s essential that employees have the skills and knowledge they need to adapt and excel.
This may involve:
- Providing regular training and workshops on quality management techniques
- Encouraging employees to pursue certifications and continuing education opportunities
- Fostering a culture of learning and knowledge sharing
- Empowering employees to take ownership of quality in their work
By investing in their people and providing them with the tools and resources they need to succeed, organizations can build a strong foundation for quality management excellence.
Case Study: IBM’s Cognitive QMS
IBM’s Cognitive QMS, powered by Watson AI, is a prime example of how advanced data management can revolutionize quality management. This innovative system integrates with existing QMS processes and provides intelligent insights to help manufacturers optimize quality, efficiency, and compliance.
Key Features and Benefits
Cognitive Process Optimization: Watson AI analyzes vast amounts of structured and unstructured data to identify patterns, anomalies, and opportunities for improvement.
Predictive Quality Insights: By leveraging machine learning algorithms, the system can predict potential quality issues and provide recommendations for preventive action.
Compliance and Risk Management: IBM’s Cognitive QMS helps manufacturers ensure compliance with industry standards and regulations, while also identifying and mitigating potential risks.
Real-World Results
In a case study published by IBM, a leading electronics manufacturer implemented Cognitive QMS and achieved remarkable results:
- 25% reduction in defects
- 15% improvement in overall equipment effectiveness
- 30% reduction in quality management costs
Overcoming Obstacles and Achieving Success
Implementing a data-driven Quality Management System is not without its challenges. Some common obstacles organizations may face include:
- Resistance to change: Employees may be hesitant to adopt new technologies or processes, particularly if they are comfortable with the status quo.
- Integration issues: Integrating new systems with existing infrastructure can be complex and time-consuming.
- Data quality concerns: Ensuring the accuracy, completeness, and reliability of data is essential for effective decision-making.
To overcome these obstacles and achieve success, organizations must:
- Start small and scale gradually: Begin with pilot projects or targeted implementations, and gradually expand as success is demonstrated and lessons are learned.
- Provide adequate training and support: Ensure that employees have the skills and resources they need to effectively use new technologies and processes.
- Continuously monitor and optimize: Regularly assess the performance of the QMS, and make adjustments as needed to ensure ongoing effectiveness and efficiency.
How CADDi Drawer Can Help in the QMS
The future of QMS is bright, and the potential for transformation is limitless. By leveraging the power of advanced data management, fostering a culture of continuous improvement, and investing in the development of their people, manufacturers can achieve new levels of quality, efficiency, and customer satisfaction.
CADDi Drawer is one of the solutions that support such data management. By using this solution, you can:
- Centralized management of documents and data: It breaks down siloed data structures and allows you to link supplier data, purchasing data, quality data, and various document groups related to QMS by using drawings, which are a shared medium throughout the manufacturing value chain, as a key.
- Make information in documents and drawings searchable: With its unique OCR algorithm, it can read and keyword-search text within documents such as SOPs, Work Instructions, Quality reports, and even footnotes in drawings.

These features significantly reduce the time spent searching for past data, allowing you to focus more on essential tasks. It also prevents overlooking necessary information or past lessons and missing out on collaboration. By doing so, it ensures the reliable execution of continuous improvement.
As you embark on your own journey to revolutionize your QMS, remember that success is not just about adopting new technologies or processes. It’s about fundamentally changing the way you think about quality and embracing a culture of excellence.
So, ask yourself:
- What steps can you take today to harness the power of data and drive meaningful change in your organization?
- How can you empower your employees to take ownership of quality and continuously improve?
- What investments can you make in training and development to build a strong foundation for success?
The answers to these questions will guide you on your path to a more intelligent, adaptive, and effective Quality Management System. The future is here, and the time to act is now.
Ready to see CADDi Drawer in action? Get a personalized demo.
Related Resources

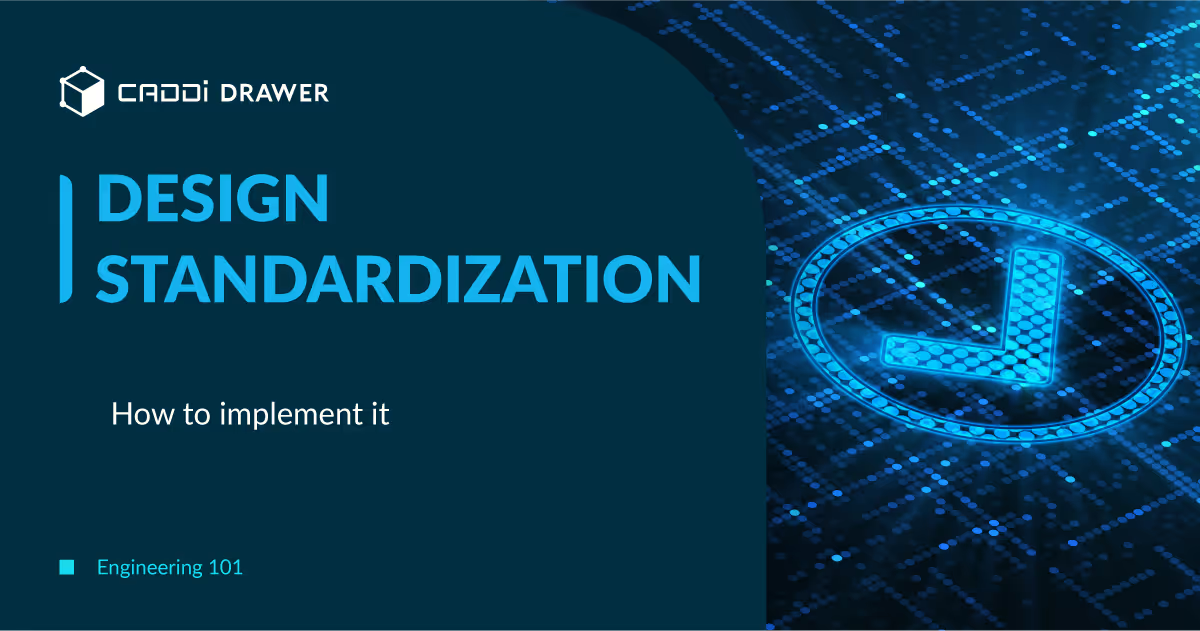
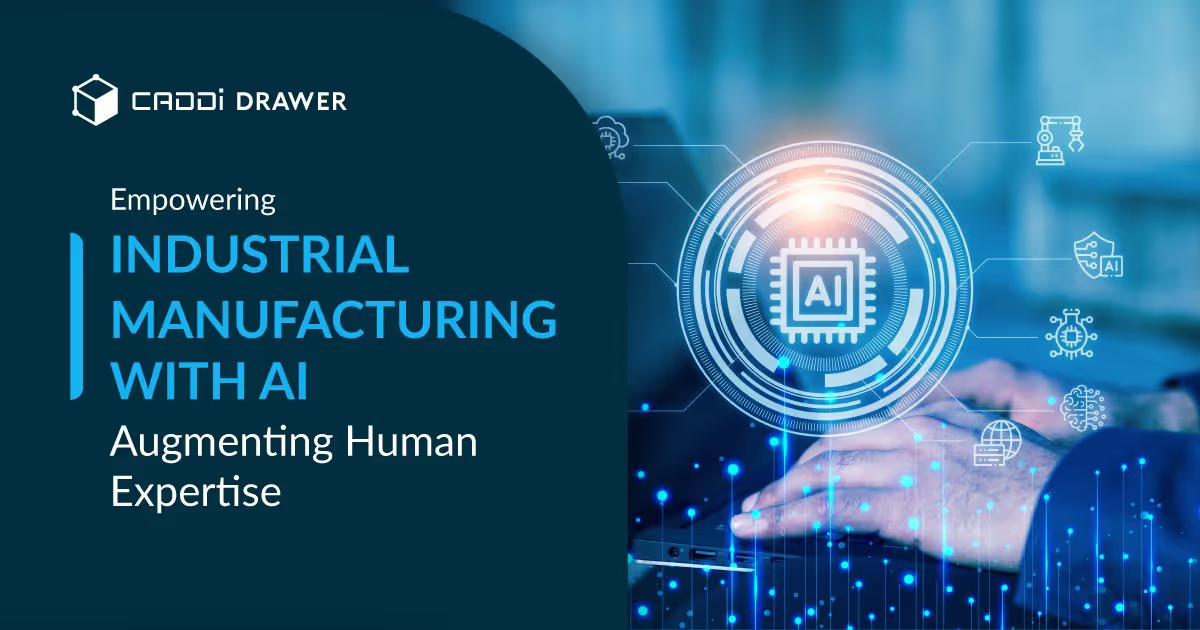
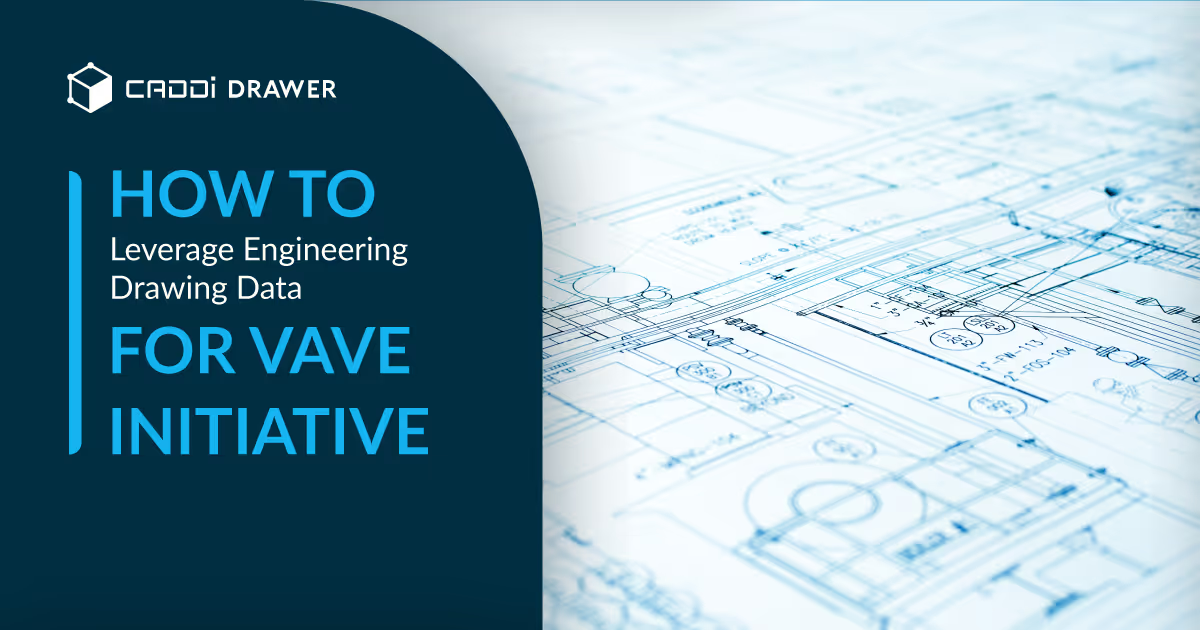
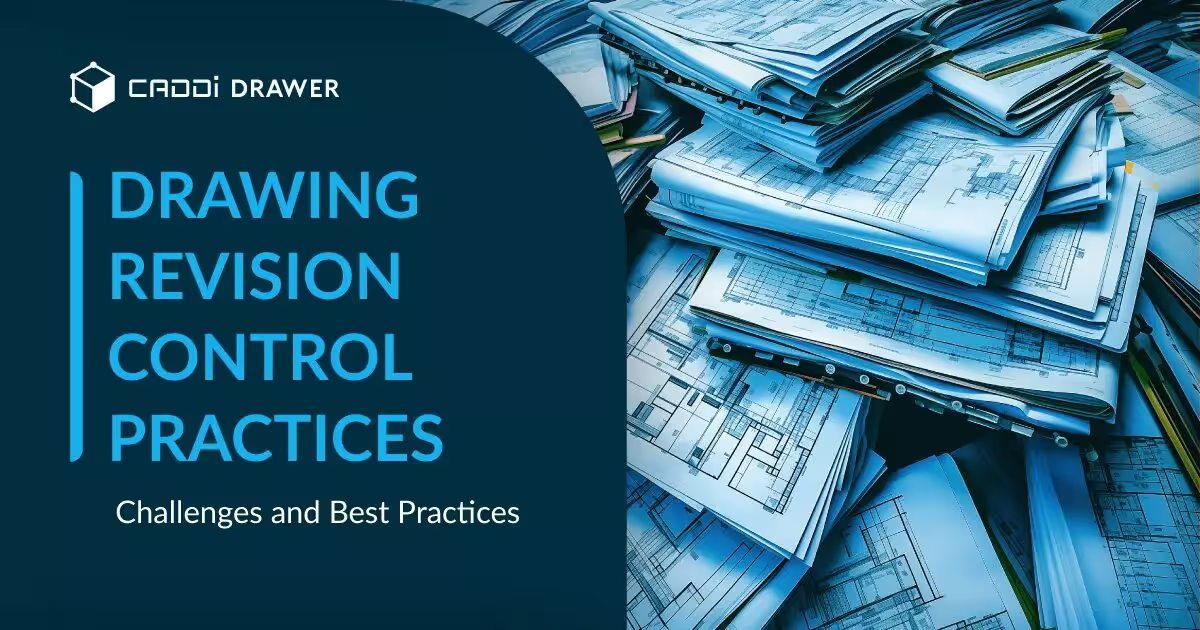
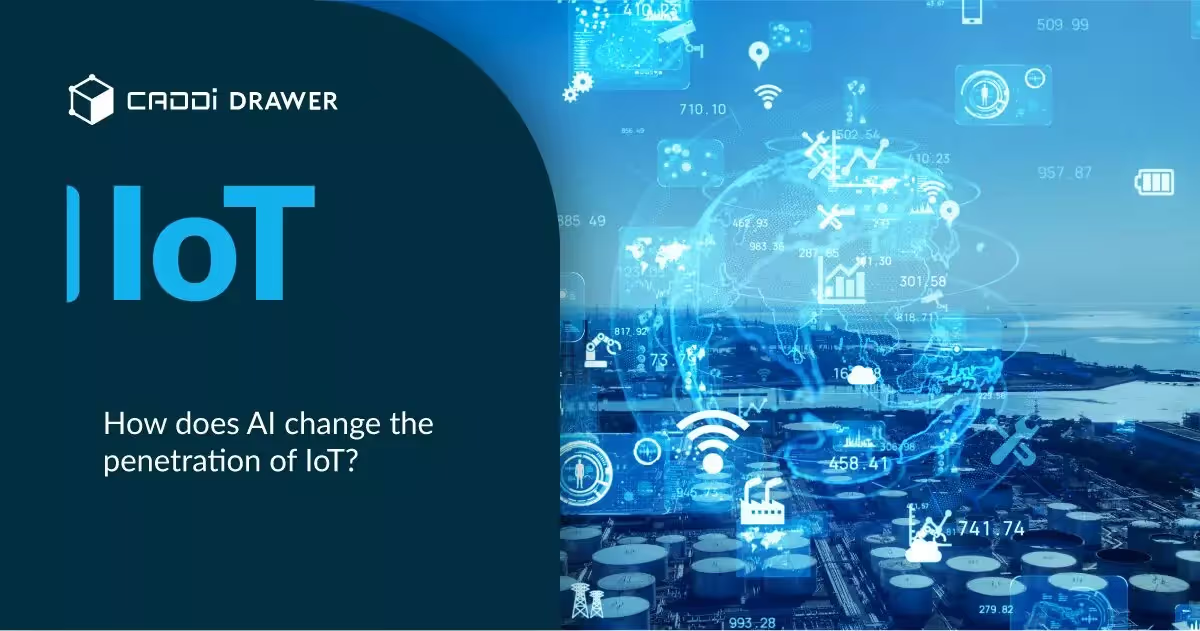