Procurement 101: Global Supply Chain Management – How it’s transforming

Table of Contents
Introduction
The term “supply chain management” (SCM), used in global supply chain management, was first coined by Keith Oliver, a consultant at Booz Allen Hamilton, in 1982. Oliver used the term to describe the process of planning, implementing, and controlling the operations of the supply chain with the purpose of satisfying customer requirements as efficiently as possible. Over the past 40 years, SCM has grown from this initial concept into a complex discipline that is crucial to the success of manufacturing and other industries.
A Brief History of Global Supply Chain Management
In the 1990s and early 2000s, the focus of SCM was on globalization, outsourcing, and lean manufacturing. Companies looked to reduce costs by sourcing materials and labor from low-cost countries and implementing just-in-time inventory practices. The development of ERP software during this time period also gave companies powerful new tools for managing their supply chains.
The 2010s brought an increased focus on risk management and resilience, as events like the 2011 Tohoku earthquake and tsunami in Japan highlighted the vulnerability of global supply chains to disruptions. This decade also saw the early adoption of digital technologies like sensors, cloud computing and advanced analytics in SCM.
Today, supply chains are more complex and face greater uncertainty than ever before, due to factors like the Covid-19 pandemic, geopolitical tensions, and the accelerating pace of technological change. In this environment, advanced digital technologies have become essential tools for supply chain professionals seeking to navigate risks and drive innovation.
The Impact of New Technologies on global supply chain management
Some of the most promising digital technologies poised to reshape supply chain management in the coming years include large language models (LLMs), generative AI, unstructured data utilization, and retrieval augmented generation (RAG).
LLMs
LLMs, like GPT, are AI systems trained on vast amounts of text data, giving them the ability to understand and generate human language with a high degree of fluency and coherence. In the supply chain context, LLMs could be used to automatically process and extract insights from the huge volumes of unstructured data that supply chains generate, such as emails, documents, drawings, photos, videos, sensor readings, and individual data across various industries. This could give supply chain professionals real-time visibility into events that may impact their operations, further enhancing global supply chain management.
Generative AI
Generative AI refers to AI systems that can create new content, such as text, images, and even physical designs, from scratch based on learned patterns. In SCM, generative AI can create initial drafts of more complex documents like contracts, RFQs, and audit reports, which stakeholders then review and refine. This “first draft” automation could make the communication process more efficient.
Unstructured data utilization
Unstructured data, which includes things like text, images, audio, and video, makes up the vast majority of global supply chain management data but has traditionally been difficult to incorporate into global supply chain management systems and processes. However, new tools like Unstructured.io enable users to extract information from files that include various unstructured data and transform them into a format that LLMs can read and understand. By analyzing unstructured data at scale, supply chain professionals unlock new insights that were previously hidden, such as emerging trends and risks.
Retrieval augmented generation (RAG)
Retrival augmented generation is an AI technique that combines the strengths of LLMs with traditional knowledge bases and information retrieval systems. RAG systems can take a query, retrieve relevant information from a knowledge base, and then use that information to generate a response using language models. In the supply chain context, RAG could be used to build powerful question answering and decision support systems that could quickly provide supply chain professionals with the information and insights they need to make better decisions.
Envisioning the Global Supply Chain of the Future
As these technologies mature and are integrated into global supply chain management, we can expect to see significant changes in how supply chains operate and the kinds of results they are able to achieve. Here are a few possible use cases that illustrate the potential of these technologies:
AI-powered global supply chain collaboration
Generative AI has the potential to streamline and automate certain types of routine communication. For example, instead of a supplier representative manually composing an email to notify a customer of a shipment delay, a generative AI system could automatically draft this message based on the relevant global supply chain management data, such as order information and logistics status.
A RAG-based system serves as a “digital supply chain assistant”, answering questions and providing guidance to supply chain professionals across multiple organizations in a supply network. By leveraging its vast knowledge base and language understanding capabilities, the system enables seamless collaboration and problem-solving, even among partners with different systems and data structures. This leads to faster issue resolution, improved decision making, and a more agile and aligned supply chain ecosystem.
Automated analysis and document creation
LLMs and generative AI combined with RAG would be able to utilize vast amounts of unstructured data, such as supplier catalogs, price lists, performance reviews, and market intelligence reports. Using natural language processing and machine learning, it identifies patterns, trends, and insights that would be difficult for humans to spot across such a large and diverse dataset.
The AI system would automate spend analysis, identify savings opportunities, and generate supplier shortlists based on multiple criteria. It creates comparative supplier profiles, simulates scenarios, and predicts impacts on key metrics. Throughout the process, the AI interacts with human decision makers via a conversational interface, ensuring insights align with business goals.
Automated risk sensing and mitigation
An AI system continuously monitors a wide range of unstructured data sources, from news articles and social media to weather reports and sensor data. Using natural language processing and machine learning, it identifies potential risks and disruptions to the supply chain, such as natural disasters, labor strikes, or supplier bankruptcies. The system would then automatically generates contingency plans and recommendations for how to mitigate those risks, which are provided to supply chain leaders through a conversational interface.
Predictive AI for demand and supply matching
An AI system ingests data on customer demand patterns, supplier lead times, logistics constraints, inventory levels, and more to generate highly accurate forecasts of future supply requirements. It then matches those forecasts against production capacity and component availability across the supply chain to predict future imbalances and recommend corrective actions in real-time. This allows the company to dynamically optimize inventory levels, production schedules, and logistics to meet customer demand while minimizing cost and risk.
Conclusion
As these examples show, the convergence of LLMs, generative AI, unstructured data utilization, and retrieval augmented generation has the potential to fundamentally transform how supply chains work. While the specific applications will vary, the unifying theme is a supply chain that is more intelligent, adaptive, and resilient – one that can sense and respond to change in real-time, and that augments and empowers human decision makers rather than replacing them.
Of course, realizing this vision will require more than just technology. It will also require changes in organizational culture, processes, and skills. Companies will need to become more data-driven and agile, and to embrace a mindset of continuous learning and experimentation. They will also need to invest in new talent and upskilling programs to build the capabilities needed to work effectively with these new technologies.
Despite these challenges, the potential benefits are simply too great to ignore. As the pace of change continues to accelerate and the business environment becomes ever more complex and uncertain, the companies that are able to harness the power of these new technologies will be the ones that thrive in the years ahead. The future of supply chain management is already here – now it’s up to forward-thinking leaders to seize the opportunity and create the supply chains of tomorrow.
Introduction
The term “supply chain management” (SCM), used in global supply chain management, was first coined by Keith Oliver, a consultant at Booz Allen Hamilton, in 1982. Oliver used the term to describe the process of planning, implementing, and controlling the operations of the supply chain with the purpose of satisfying customer requirements as efficiently as possible. Over the past 40 years, SCM has grown from this initial concept into a complex discipline that is crucial to the success of manufacturing and other industries.
A Brief History of Global Supply Chain Management
In the 1990s and early 2000s, the focus of SCM was on globalization, outsourcing, and lean manufacturing. Companies looked to reduce costs by sourcing materials and labor from low-cost countries and implementing just-in-time inventory practices. The development of ERP software during this time period also gave companies powerful new tools for managing their supply chains.
The 2010s brought an increased focus on risk management and resilience, as events like the 2011 Tohoku earthquake and tsunami in Japan highlighted the vulnerability of global supply chains to disruptions. This decade also saw the early adoption of digital technologies like sensors, cloud computing and advanced analytics in SCM.
Today, supply chains are more complex and face greater uncertainty than ever before, due to factors like the Covid-19 pandemic, geopolitical tensions, and the accelerating pace of technological change. In this environment, advanced digital technologies have become essential tools for supply chain professionals seeking to navigate risks and drive innovation.
The Impact of New Technologies on global supply chain management
Some of the most promising digital technologies poised to reshape supply chain management in the coming years include large language models (LLMs), generative AI, unstructured data utilization, and retrieval augmented generation (RAG).
LLMs
LLMs, like GPT, are AI systems trained on vast amounts of text data, giving them the ability to understand and generate human language with a high degree of fluency and coherence. In the supply chain context, LLMs could be used to automatically process and extract insights from the huge volumes of unstructured data that supply chains generate, such as emails, documents, drawings, photos, videos, sensor readings, and individual data across various industries. This could give supply chain professionals real-time visibility into events that may impact their operations, further enhancing global supply chain management.
Generative AI
Generative AI refers to AI systems that can create new content, such as text, images, and even physical designs, from scratch based on learned patterns. In SCM, generative AI can create initial drafts of more complex documents like contracts, RFQs, and audit reports, which stakeholders then review and refine. This “first draft” automation could make the communication process more efficient.
Unstructured data utilization
Unstructured data, which includes things like text, images, audio, and video, makes up the vast majority of global supply chain management data but has traditionally been difficult to incorporate into global supply chain management systems and processes. However, new tools like Unstructured.io enable users to extract information from files that include various unstructured data and transform them into a format that LLMs can read and understand. By analyzing unstructured data at scale, supply chain professionals unlock new insights that were previously hidden, such as emerging trends and risks.
Retrieval augmented generation (RAG)
Retrival augmented generation is an AI technique that combines the strengths of LLMs with traditional knowledge bases and information retrieval systems. RAG systems can take a query, retrieve relevant information from a knowledge base, and then use that information to generate a response using language models. In the supply chain context, RAG could be used to build powerful question answering and decision support systems that could quickly provide supply chain professionals with the information and insights they need to make better decisions.
Envisioning the Global Supply Chain of the Future
As these technologies mature and are integrated into global supply chain management, we can expect to see significant changes in how supply chains operate and the kinds of results they are able to achieve. Here are a few possible use cases that illustrate the potential of these technologies:
AI-powered global supply chain collaboration
Generative AI has the potential to streamline and automate certain types of routine communication. For example, instead of a supplier representative manually composing an email to notify a customer of a shipment delay, a generative AI system could automatically draft this message based on the relevant global supply chain management data, such as order information and logistics status.
A RAG-based system serves as a “digital supply chain assistant”, answering questions and providing guidance to supply chain professionals across multiple organizations in a supply network. By leveraging its vast knowledge base and language understanding capabilities, the system enables seamless collaboration and problem-solving, even among partners with different systems and data structures. This leads to faster issue resolution, improved decision making, and a more agile and aligned supply chain ecosystem.
Automated analysis and document creation
LLMs and generative AI combined with RAG would be able to utilize vast amounts of unstructured data, such as supplier catalogs, price lists, performance reviews, and market intelligence reports. Using natural language processing and machine learning, it identifies patterns, trends, and insights that would be difficult for humans to spot across such a large and diverse dataset.
The AI system would automate spend analysis, identify savings opportunities, and generate supplier shortlists based on multiple criteria. It creates comparative supplier profiles, simulates scenarios, and predicts impacts on key metrics. Throughout the process, the AI interacts with human decision makers via a conversational interface, ensuring insights align with business goals.
Automated risk sensing and mitigation
An AI system continuously monitors a wide range of unstructured data sources, from news articles and social media to weather reports and sensor data. Using natural language processing and machine learning, it identifies potential risks and disruptions to the supply chain, such as natural disasters, labor strikes, or supplier bankruptcies. The system would then automatically generates contingency plans and recommendations for how to mitigate those risks, which are provided to supply chain leaders through a conversational interface.
Predictive AI for demand and supply matching
An AI system ingests data on customer demand patterns, supplier lead times, logistics constraints, inventory levels, and more to generate highly accurate forecasts of future supply requirements. It then matches those forecasts against production capacity and component availability across the supply chain to predict future imbalances and recommend corrective actions in real-time. This allows the company to dynamically optimize inventory levels, production schedules, and logistics to meet customer demand while minimizing cost and risk.
Conclusion
As these examples show, the convergence of LLMs, generative AI, unstructured data utilization, and retrieval augmented generation has the potential to fundamentally transform how supply chains work. While the specific applications will vary, the unifying theme is a supply chain that is more intelligent, adaptive, and resilient – one that can sense and respond to change in real-time, and that augments and empowers human decision makers rather than replacing them.
Of course, realizing this vision will require more than just technology. It will also require changes in organizational culture, processes, and skills. Companies will need to become more data-driven and agile, and to embrace a mindset of continuous learning and experimentation. They will also need to invest in new talent and upskilling programs to build the capabilities needed to work effectively with these new technologies.
Despite these challenges, the potential benefits are simply too great to ignore. As the pace of change continues to accelerate and the business environment becomes ever more complex and uncertain, the companies that are able to harness the power of these new technologies will be the ones that thrive in the years ahead. The future of supply chain management is already here – now it’s up to forward-thinking leaders to seize the opportunity and create the supply chains of tomorrow.
Ready to see CADDi Drawer in action? Get a personalized demo.
Subscribe to our Blog!
Related Resources




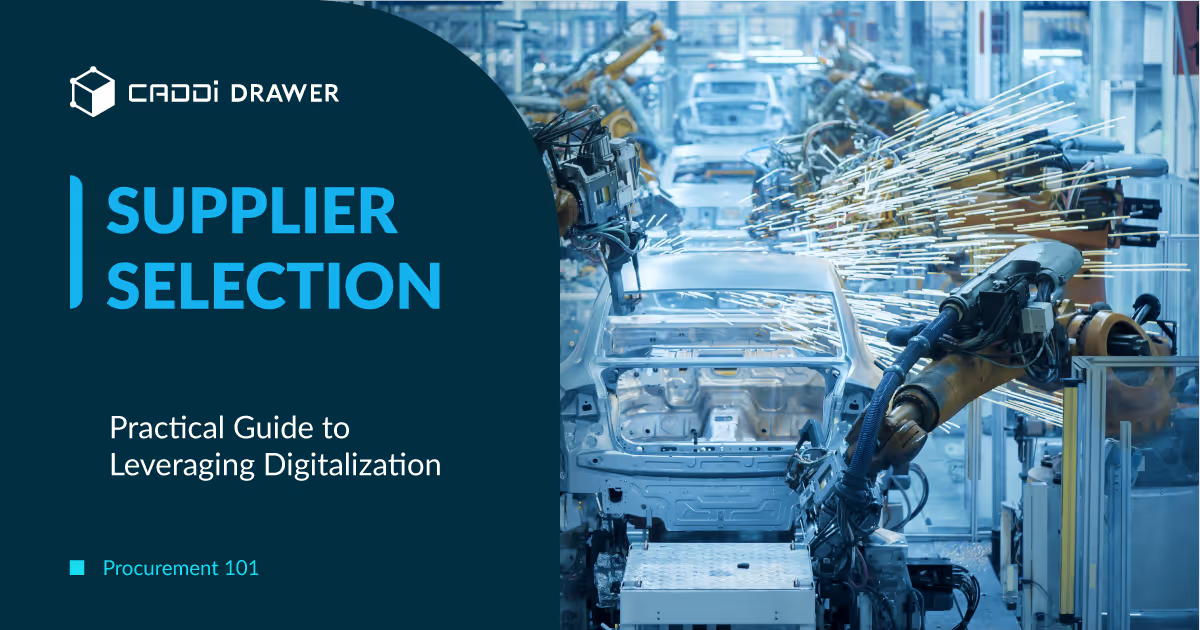
